2 Background Removal with SVD - DRAFT π
based on: original Fast.ai notebook
2.1 Setup
We use a few of the Noj underlying libraries, clj-media, and Apache Commons Math.
ns svd
(:require [tablecloth.api :as tc]
(:as clj-media]
[com.phronemophobic.clj-media :as clj-media.model]
[com.phronemophobic.clj-media.model :as dtype]
[tech.v3.datatype :as tensor]
[tech.v3.tensor :as bufimg]
[tech.v3.libs.buffered-image :as kind]
[scicloj.kindly.v4.kind :as mat]
[fastmath.matrix :as dfn]
[tech.v3.datatype.functional :as dstats])
[tech.v3.datatype.statistics :import (org.apache.commons.math3.linear
( SingularValueDecomposition)))
2.2 Reading a video file
We downloaded the following file from the original notebook. It seems to be a shorter version of the full original video (just the first 50 seconds).
def video-path
("notebooks/movie/Video_003.mp4")
(kind/video:src video-path}) {
Let us explore it with clj-media:
(clj-media/probe video-path)
:streams
{:width 320,
[{:num-frames 350,
:stream-index 0,
:codec
:name "h264",
{:long-name "H.264 / AVC / MPEG-4 AVC / MPEG-4 part 10",
:media-type :media-type/video,
:id 27},
:bit-rate 169285,
:video-delay 0,
:pixel-format :pixel-format/yuv420p,
:estimated-duration 716800,
:time-base [1 14336],
:height 240,
:media-type :media-type/video}]}
2.3 Converting the video to tensor structures
Using clj-media, we can reduce over frames:
(clj-media/frames
(clj-media/file video-path):video
:format (clj-media/video-format
{:pixel-format
{:pixel-format/rgba})})
filter.media.FramesReducible 0xae4d3ef "com.phronemophobic.clj_media.impl.filter.media.FramesReducible@ae4d3ef"] #object[com.phronemophobic.clj_media.impl.
For example, let us extract the first frame and convert it to an image:
def first-image
(first
(into []
(comp (take 1)
(map clj-media.model/image))
(
(clj-media/frames
(clj-media/file video-path):video
:format (clj-media/video-format
{:pixel-format
{:pixel-format/rgba})}))))
first-image
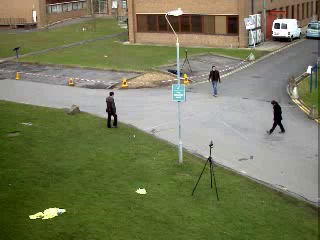
When converting to a tensor, we have the four color components of rgba
format:
(bufimg/as-ubyte-tensor first-image)
240 320 4]
#tech.v3.tensor<uint8>[255 105 137 169]
[[[255 105 137 169]
[255 105 137 169]
[
...255 45 59 85]
[255 37 55 75]
[255 30 48 68]]
[255 105 137 169]
[[255 105 137 169]
[255 105 137 169]
[
...255 11 25 51]
[255 22 40 60]
[255 24 42 62]]
[255 105 137 169]
[[255 105 137 169]
[255 105 137 169]
[
...255 15 29 55]
[255 28 46 66]
[255 31 49 69]]
[
...255 0 45 31]
[[255 0 46 32]
[255 0 47 33]
[
...255 22 76 63]
[255 3 57 44]
[255 0 54 41]]
[255 0 48 34]
[[255 0 47 33]
[255 0 46 32]
[
...255 24 78 65]
[255 6 60 47]
[255 2 56 43]]
[255 3 53 39]
[[255 2 52 38]
[255 0 49 35]
[
...255 22 76 63]
[255 3 57 44]
[255 0 54 41]]] [
In our case, the first component (a) is fixed:
-> (let [t (bufimg/as-ubyte-tensor first-image)]
(240 320]
(tensor/compute-tensor [fn [i j]
(0))
(t i j :uint8))
dtype/->bufferdistinct)
255) (
The rgb components are the other three.
We wish to process all frames, but resize the images to a lower resolution, and turn them to gray-scale.
See Luma for discussion of the gray-scale formula: 0.299 β Red + 0.587 β Green + 0.114 β Blue
defn image->small-tensor [image]
(let [w 160
(120
h -> image
t (
(bufimg/resize w h {})
bufimg/as-ubyte-tensor)]
(tensor/compute-tensor [h w]fn [i j]
(+ (* 0.299 (t i j 1))
(* 0.587 (t i j 2))
(* 0.113 (t i j 3))))
(:uint8)))
-> first-image
(
image->small-tensor bufimg/tensor->image)
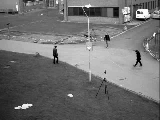
Now let us collect the small tensors. We will take just 70 frames of the video, simply because it is enough for demonstrating the methods of this tutorial.
def small-tensors
(into []
(comp (take 70)
(map (comp image->small-tensor
(
clj-media.model/image)))
(clj-media/frames
(clj-media/file video-path):video
:format (clj-media/video-format
{:pixel-format
{:pixel-format/rgba})})))
count small-tensors) (
70
2.4 Reshaping the data
Now we will reshape the data as one matrix with row per pixel and column per frame.
def flat-tensors
(->> small-tensors
(mapv dtype/->buffer))) (
def long-tensor
(-> flat-tensors first count)
(tensor/compute-tensor [(count flat-tensors)]
(fn [j i]
(
((flat-tensors i) j)):uint8))
(kind/hiccup:div
[:h3 "It is interesting to scroll this! π"]
[:div {:style {:max-height "400px"
[:overflow :auto}}
(bufimg/tensor->image long-tensor)]])
It is interesting to scroll this! π

2.5 Singular value decomposition
Let us now compute the SVD.
We can use Fastmathβs matrix API to convert out structures to the RealMatrix type of Apache Commons Math.
def matrix
(->> long-tensor
(map double-array)
( (mat/rows->RealMatrix)))
def svd
( (SingularValueDecomposition. matrix))
(.getSingularValues svd)
139195.94251037698, 6275.043162591476, 5603.154539852162,
[5033.876530323665, 4748.4364541889845, 4466.280602711257,
3925.463833639647, 3422.0744858435796, 3342.4862210577885,
3082.423188126279, 2929.5173315995453, 2793.49945570757,
2717.285832811692, 2666.1808760367185, 2541.216199664794,
2432.7718341294694, 2319.3149114242406, 2120.269146998713,
2081.0267854207323, 1980.0303983996318, 1876.8205337895033,
1762.5960795337005, 1699.5845163689771, 1656.5508404583634,
1609.9675860772804, 1483.3874664237662, 1446.215438829954,
1382.8701991961054, 1364.4240301460377, 1350.942042502351,
1277.4312844397282, 1228.5910914812407, 1222.3556128225734,
1197.89073832447, 1169.7744044718058, 1127.928042628131,
1095.499471859092, 1085.6859462873324, 1066.7857900518645,
1062.0508770324302, 1024.6891477000197, 1006.3974197040208,
995.327635131667, 969.9391120995942, 954.8477254064976,
930.2979788456232, 916.5794865562222, 906.7089686677912,
885.1719689660408, 880.5136645699995, 857.8187666956718,
845.8275784138032, 834.536795218182, 811.374667317597,
800.2167044812257, 789.4260520421147, 771.865842648705,
752.1605409807378, 744.6758461913287, 720.2939212922001,
703.9227223493441, 696.5407308135284, 693.0005788642618,
686.4606585523608, 665.788536654086, 654.9844812376992,
651.0476161470714, 626.1163848501176, 584.2795813666896,
553.9183283855124]
def shape
(juxt mat/nrow
( mat/ncol))
(shape (.getU svd))
19200 70] [
(shape (.getS svd))
70 70] [
(shape (.getVT svd))
70 70] [
To visualize different parts of the matrix decomposition, we will need to normalize tensors to the [0,1] range:
defn tensor-normalize
(
[t]let [{:keys [min max]} (dstats/descriptive-statistics
(
t:min :max})]
#{-> (dfn/- t min)
(- (double max) (double min)))))) (dfn// (
For example:
-> [[1 2 3]
(4 5 6]]
[
tensor/->tensor tensor-normalize)
2 3]
#tech.v3.tensor<object>[0.000 0.2000 0.4000]
[[ 0.6000 0.8000 1.000]] [
Now let us visualize the main component of our matrix.
def component0
(let [i 0]
(-> (.getColumnMatrix (.getU svd) i)
(nth (.getSingularValues svd)
(mat/muls (
i)) (mat/mulm (.getRowMatrix (.getVT svd) i)))))
(shape component0)
19200 70] [
This is the first order approximation of the pixel-by-frame matrix by the SVD method.
Let us take its first column, which is the first frame, and show it as an image:
defn matrix->first-image [m]
(-> m
(0)
(.getColumn
dtype/->array-buffer
tensor-normalize255)
(dfn/*
(dtype/->int-array)120 160])
(tensor/reshape [ bufimg/tensor->image))
defn matrix->first-image [m]
(-> m
(0)
(.getColumn
dtype/->array-buffer
tensor-normalize255)
(dfn/*
(dtype/->int-array)120 160])
(tensor/reshape [ bufimg/tensor->image))
(matrix->first-image component0)
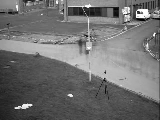
We see it is the background image of the video.
Now let us compute the remainder after removing the first component.
def residual
(
(mat/sub matrix component0))
(matrix->first-image residual)
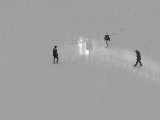
We see these are the people.
2.6 Visualizing the decomposition with the first image:
Let us summarize the decomposition:
->> [matrix
(
component0
residual]mapv matrix->first-image)) (
[
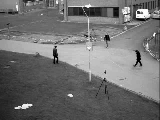
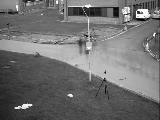
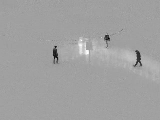
]
2.7 Generating decomposed videos
defn matrix->images [m]
(-> m
(
mat/mat->array
dtype/->array-buffer
tensor-normalize255)
(dfn/*
dtype/->int-array120 160 70])
(tensor/reshape [2 0 1])
(tensor/transpose [->> (mapv bufimg/tensor->image)))) (
->> residual
(
matrix->imagestake 20)) (
(
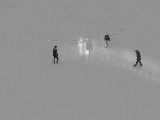
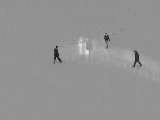
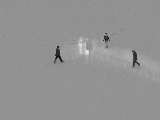
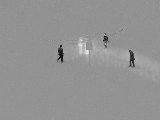
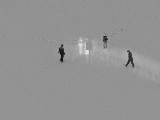
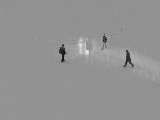
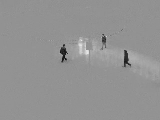
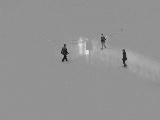
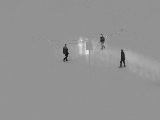
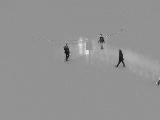
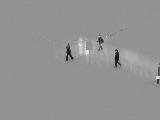
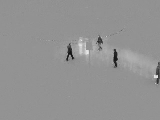
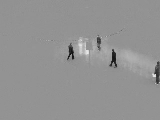
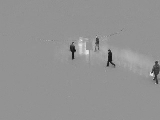
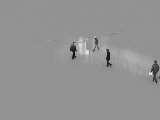
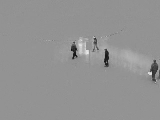
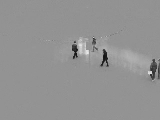
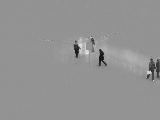
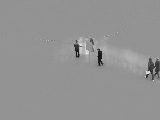
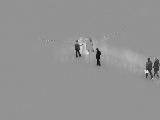
)
def frame-format
(:pixel-format :pixel-format/gray8
(clj-media/video-format {:time-base 7
:line-size 160
:width 160
:height 120}))
defn img->frame [img pts time-base]
(
(clj-media/make-frame:bytes (-> img
{
(.getData)
(.getDataBuffer)
(.getData)):format frame-format
:time-base time-base
:pts pts}))
def generated-frames
(let [frame-rate 7
(10
seconds * seconds frame-rate)]
num-frames (into []
(fn [pts image]
(map-indexed (
(img->frame image pts frame-rate))) (matrix->images residual))))
def target-path
("notebooks/generated-movie.mp4")
(clj-media/write!
(clj-media/make-media frame-format generated-frames) target-path)
nil
(kind/video:src target-path}) {